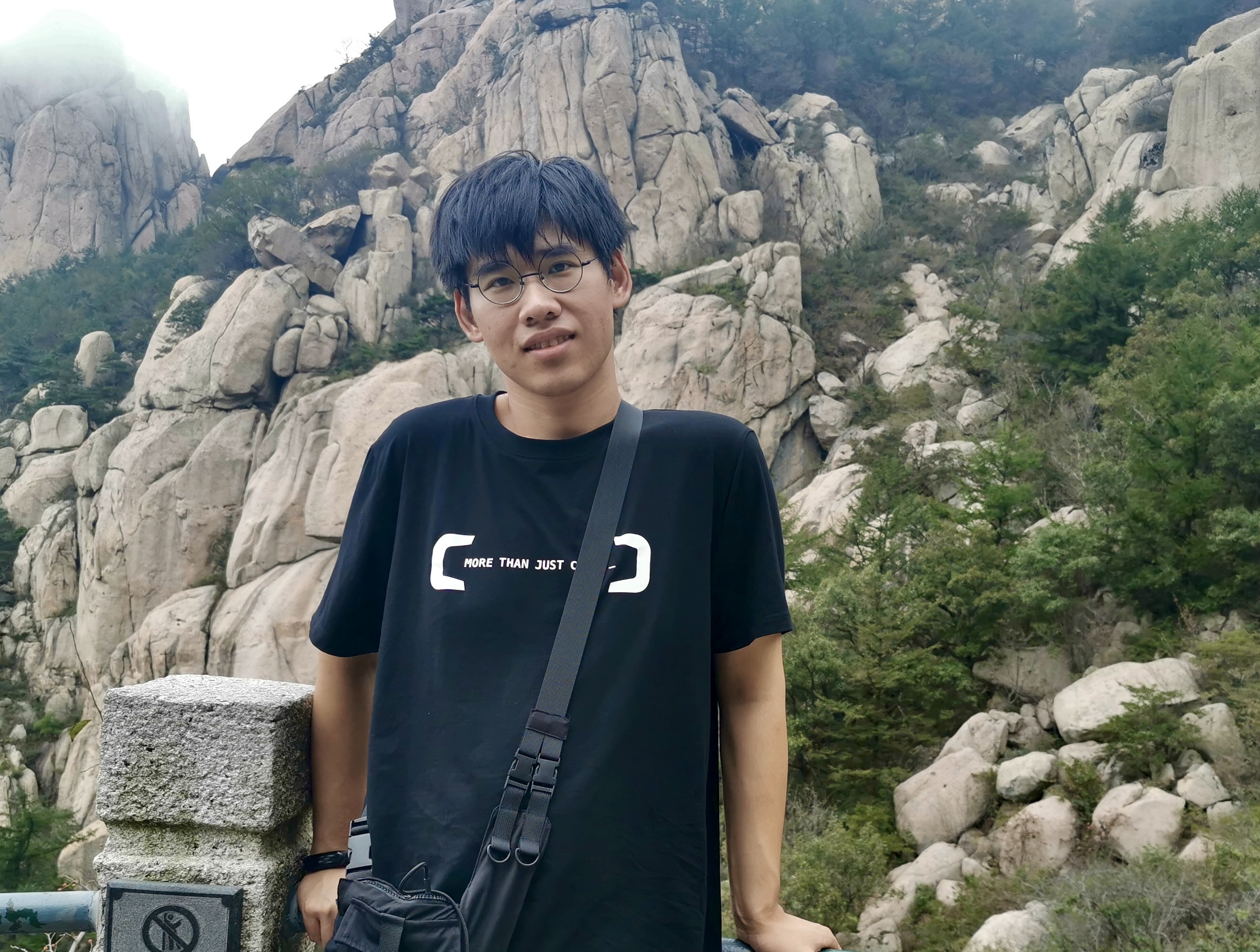
Xiaoze Liu
ねだるな、勝ち取れ、さすれば与えられん
# About
I'm a PhD student at Purdue University, advised by Prof. Jing Gao and Prof. Xiaoqian Wang. I hold a Master's Degree with honors from Zhejiang University (China) and a Bachelor's Degree with honors from Northeastern University (China).
# News
[Jul 2025] SUV, a scalable copyright compliance framework for large language models (LLMs) with regularized selective unlearning, is accepted by COLM 2025.
[May 2025] I joined AWS AI Labs – Fundamental Research Team as an Applied Scientist Intern for Summer 2025.
[May 2025] I will present a portion of the tutorial, LLMs and Copyright Risks: Benchmarks and Mitigation Approaches, at NAACL 2025.
# Recent Publications
SUV: Scalable Large Language Model Copyright Compliance with Regularized Selective Unlearning Tianyang Xu#, Xiaoze Liu#, Feijie Wu, Xiaoqian Wang, Jing Gao, The 2025 Conference on Language Modeling (COLM), 2025.
SHIELD: Evaluation and Defense Strategies for Copyright Compliance in LLM Text Generation. Xiaoze Liu#, Ting Sun#, Tianyang Xu, Feijie Wu, Cunxiang Wang, Xiaoqian Wang, Jing Gao, The 2024 Conference on Empirical Methods in Natural Language Processing (EMNLP), 2024.
Survey on Factuality in Large Language Models: Knowledge, Retrieval and Domain-Specificity. Cunxiang Wang#, Xiaoze Liu#, Yuanhao Yue#, Qipeng Guo, Xiangkun Hu, Xiangru Tang, Tianhang Zhang, Jiayang Cheng, Yunzhi Yao, Wenyang Gao, Xuming Hu, Zehan Qi, Yidong Wang, Linyi Yang, Jindong Wang, Xing Xie, Zheng Zhang, Yue Zhang, ACM Computing Surveys (CSUR), 2025
Towards Federated RLHF with Aggregated Client Preference for LLMs. Feijie Wu, Xiaoze Liu, Haoyu Wang, Xingchen Wang, Lu Su, Jing Gao, The Thirteenth International Conference on Learning Representations (ICLR), 2025
Evaluating the Factuality of Large Language Models using Large-Scale Knowledge Graphs. Xiaoze Liu, Feijie Wu, Tianyang Xu, Zhuo Chen, Yichi Zhang, Xiaoqian Wang, Jing Gao IEEE Data Engineering Bulletin, 2024
SaySelf: Teaching LLMs to Express Confidence with Self-Reflective Rationales. EMNLP 2024 Tianyang Xu, Shujin Wu, Shizhe Diao, Xiaoze Liu, Xingyao Wang, Yangyi Chen, Jing Gao, The 2024 Conference on Empirical Methods in Natural Language Processing (EMNLP), 2024.
CausalEval: Towards Better Causal Reasoning in Language Models. Longxuan Yu, Delin Chen, Siheng Xiong, Qingyang Wu, Qingzhen Liu, Dawei Li, Zhikai Chen, Xiaoze Liu, Liangming Pan, The 2025 Annual Conference of the Nations of the Americas Chapter of the ACL (NAACL), 2025
...
# Education and Experience
- PhD Student, Purdue University, 2023 - Present
- Advised by Prof. Jing Gao and Prof. Xiaoqian Wang
- Applied Scientist Intern, AWS AI Labs – Fundamental Research Team, 2025
- Master's Degree, Zhejiang University, 2020 - 2023, Graduated with Outstanding Graduate Award
- Awarded Outstanding Master’s Thesis of Zhejiang Province (Top 2%)
- Research Intern, AliCloud & Alibaba DAMO Academy, 2021-2023
- Bachelor's Degree, Northeastern University, 2016 - 2020, Graduated with Outstanding Graduate Award
- Intern, ByteDance, 2019 - 2020
- Exchange Student, Kanazawa University, 2019 - 2020, Supported by JASSO Scholarship
# Academic Services
Serve as a reviewer/PC for
- 2025: KDD, ICLR, ICML, NeurIPS, ACL Rolling Review
- 2024: KDD, NeurIPS, ACM MM, SDM, CIKM, ISWC, ACL Rolling Review
- 2023: KDD, NeurIPS, EMNLP
Served as a Journal reviewer for Transactions on Machine Learning Research, IEEE Transactions on Knowledge and Data Engineering, IEEE Transactions on Neural Networks and Learning Systems, Pattern Recognition, Information Sciences, IEEE Transactions on Big Data